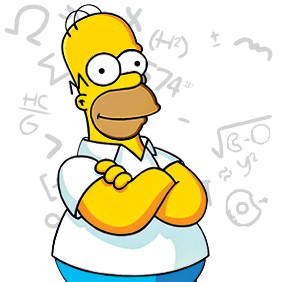
XGBoost
Python
학습 에러시 상단에 다음 설정을 추가한다.
os.environ['KMP_DUPLICATE_LIB_OK'] = 'True'
XGBoost Parameters
임의로 지정하지 말고 optuna로 튜닝해볼 것.
n_estimators=200, max_depth=3, gamma=0.5, subsample=0.5
n_estimators
트리 갯수1
gamma
[default=0, alias:min_split_loss
] Minimum loss reduction required to make a further partition on a leaf node of the tree. 리프 노드에 추가 파티션을 생성할때의 최소 손실 감소. The larger gamma is, the more conservative the algorithm will be. 감마가 클수록 알고리즘은 더 보수적이다.- range: [0,∞]
max_depth
[default=6] Maximum depth of a tree. Increasing this value will make the model more complex and more likely to overfit. 이 값을 증가시키면 모델이 더 복잡해지고 오버피팅이 발생하기 쉽다. 0 indicates no limit. Note that limit is required when grow_policy is set of depthwise. grow_policy가 depthwise(디폴트)로 설정된 경우 limit 설정이 필요하다.- range: [0,∞]
subsample
[default=1] Subsample ratio of the training instances. Setting it to 0.5 means that XGBoost would randomly sample half of the training data prior to growing trees. 0.5로 설정하면 XGBoost가 트리를 키우기 전에 학습 데이터의 절반을 임의로 샘플링한다. and this will prevent overfitting. 값이 작을수록 오버피팅을 방지한다. Subsampling will occur once in every boosting iteration.- range: (0,1]
Last Modified: 2021/06/08 13:03:45