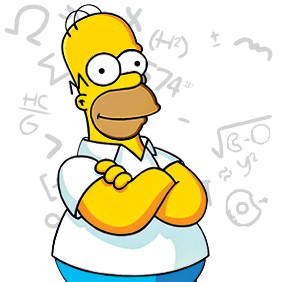
NVIDIA
NVIDIA Data Center GPUs
Name | bits/s | Bytes/s |
---|---|---|
SanDisk Extreme SSD | 550MB/s read | |
USB 3.1 Gen 2 | 10Gb/s | 1.25GB/s |
HDMI | 10Gb/s | 1.25GB/s |
HDMI 4K | 18Gb/s | 2.25GB/s |
M1 Macbook Pro 1TB SSD | 7.4GB/s read | |
M1 Pro Memory | 200GB/s | |
M1 Max Memory | 400GB/s | |
NVLink(A100) | 600GB/s | |
HBM(A100 40G) | 1.5TB/s | |
HBM(A100 80G) | 1.9TB/s |
V100, A100, H100
Streaming Multiprocessor (SM), Compute Unit (AMD)
SuperPOD
Compute Nodes: 40ea DGX A100 system(8x A100)
A100 80GB부터는 VRAM이 80GB. V100까지는 16/32GB까지만 제공되어 insufficient memory error가 잦았다.
$ nvidia-smi topo --matrix
GPU0 GPU1 GPU2 GPU3 GPU4 GPU5 GPU6 GPU7 CPU Affinity NUMA Affinity
GPU0 X NV1 NV1 NV2 NV2 PHB PHB PHB 0-63 0-1
GPU1 NV1 X NV2 NV1 PHB NV2 PHB PHB 0-63 0-1
GPU2 NV1 NV2 X NV2 PHB PHB NV1 PHB 0-63 0-1
GPU3 NV2 NV1 NV2 X PHB PHB PHB NV1 0-63 0-1
GPU4 NV2 PHB PHB PHB X NV1 NV1 NV2 0-63 0-1
GPU5 PHB NV2 PHB PHB NV1 X NV2 NV1 0-63 0-1
GPU6 PHB PHB NV1 PHB NV1 NV2 X NV2 0-63 0-1
GPU7 PHB PHB PHB NV1 NV2 NV1 NV2 X 0-63 0-1
Legend:
X = Self
SYS = Connection traversing PCIe as well as the SMP interconnect between NUMA nodes (e.g., QPI/UPI)
NODE = Connection traversing PCIe as well as the interconnect between PCIe Host Bridges within a NUMA node
PHB = Connection traversing PCIe as well as a PCIe Host Bridge (typically the CPU)
PXB = Connection traversing multiple PCIe bridges (without traversing the PCIe Host Bridge)
PIX = Connection traversing at most a single PCIe bridge
NV# = Connection traversing a bonded set of # NVLinks
Last Modified: 2024/04/29 09:55:08